Invited Speakers
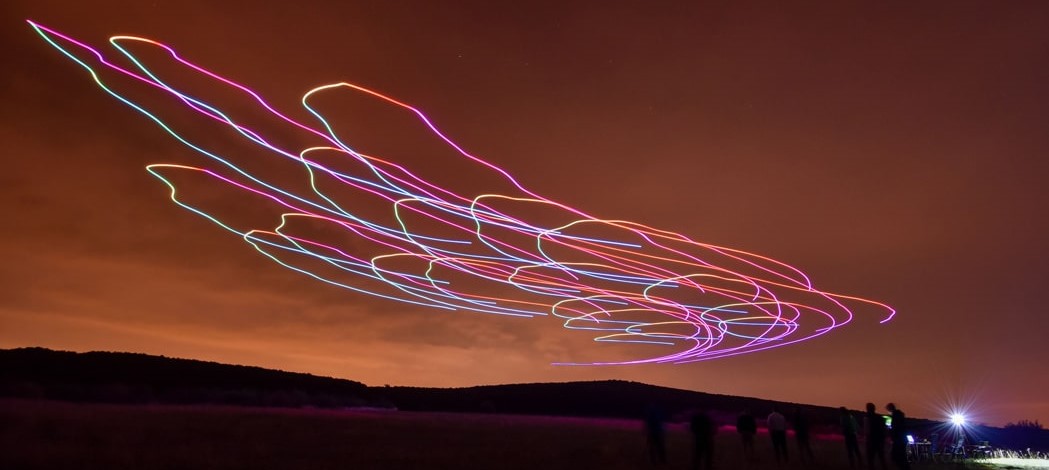
Gábor Vásárhelyi
Senior research fellow at the MTA-ELTE Statistical and Biological Physics Research Group, leader of the Robotic Lab at ELTE Department of Biological Physics and CEO of CollMot Robotics Ltd.
Talk title: Adaptive flocking with simultaneous stability and reactivity
Short bio:
Gábor Vásárhelyi is a researcher in the field of collective motion and collective behavior of animals and robots (drones). He received his MSc in engineering-physics from the Technical University of Budapest in 2003, and his PhD in technical sciences (info-bionics) from Péter Pázmány Catholic University, Hungary, in 2007. Since 2009 he is with Eötvös University, Department of Biological Physics as leader of the Robotic Lab at Tamas Vicsek’s Research Group on collective motion. He is currently a senior research fellow at MTA-ELTE Statistical and Biological Physics Research Group and CEO of CollMot Robotics Ltd., a spin-off dedicated to multi-drone services.
Talk abstract: Our lab is specialized in investigating different aspects of bio-inspired collective behavior with large simulated and real drone swarms.
One of the fundamental building blocks of collective behavior we target is flocking.
When going over 100-1000 agents in realistic state-of-the-art flocking simulations, we start to face an interesting tradeoff between tuning a system to be either overdamped, i.e. stable/persistent, or underdamped, i.e. reactive/responsive.
High persistence reduces noise-induced fluctuations, but also means that the system has some kind of inertia, which, on the other hand is disadvantageous when a quick global response (high responsivity) is needed to some internal or external stimuli, e.g. in front of walls/obstacles/predators. Following the observation that higher order species (like birds) can naturally form flocks that are both persistent and responsive, we designed a model in which we replace the egalitarian flocking rules with an adaptive hierarchical control framework. The local decisions in the flocking model are still driven by the average of neighboring decisions, but they are now weighted by the new information content agents are faced to and share actively. With this new model we can break out of the previous persistence-responsivity tradeoff and can create truly scalable flocks of up to several thousands of agents in simulation.
Co-authors: Boldizsár Balázs and Tamás Vicsek
Giuseppe Loianno
Assistant Professor in the Electrical and Computer Engineering Department and in the Mechanical and
Aerospace
Engineering Department at the Tandon School of Engineering of New York University
Talk title: Autonomous Agile Navigation and Transportation
Short bio: Prof. Giuseppe Loianno is an assistant professor at the New York University and director of the Agile Robotics and Perception Lab (https://wp.nyu.edu/arpl/) working on autonomous Micro Aerial Vehicles. Prior to NYU he was a lecturer, research scientist, and team leader at the General Robotics, Automation, Sensing and Perception (GRASP) Laboratory at the University of Pennsylvania. He received his BSc and MSc degrees in automation engineering, both with honors, from the University of Naples “Federico II” in December 2007 and February 2010, respectively. He received his PhD in computer and control engineering focusing in robotics in May 2014 in the PRISMA Lab group. Dr. Loianno has published more than 50 conference papers, journal papers, and book chapters. His research interests include visual odometry, sensor fusion, and visual servoing for micro aerial vehicles. He received the Conference Editorial Board Best Reviewer Award at ICRA 2016, National Italian American Foundation (NIAF) Young Investigator Award 2018. He is the program chair of IEEE SSRR 2019. He has organized multiple workshops on Micro Aerial Vehicles during IROS conferences and created the new International Symposium on Aerial Robotics (ISAR). His work has been featured in a large number of renowned international news and magazines.
Talk abstract: Drones are starting to play a major role in several tasks such as search and rescue, interaction with the environment, inspection, patrolling and monitoring.
Agile navigation of Micro Aerial Vehicles (MAVs) through unknown environments poses a number of challenges in terms of perception, state estimation, planning, and control.
These challenges are exacerbated when dealing and deploying swarms of drones, where the coordination and consensus in terms of planning, localization, and control among the agents become essential to guarantee the overall mission success.
To achieve this, MAVs have to localize themselves and coordinate between each other in unstructured environments. In this talk, I will present some recent research results on high speed and agile navigation using multiple drones using a minimal on-board sensor suite composed by a single camera system and IMU. I will also discuss the requirements and the additional challenges in terms of navigation and coordination once the vehicles are physically interconnected to solve a transportation tasks.
Martin Saska
Head of Multi-robot Systems Group in the Department of Cybernetics, Czech Technical University in Prague
Talk title: UV-light based stabilization of aerial swarms in real-world environments
Short bio:
Martin Saska received his MSc. degree at Czech Technical University in Prague, 2005, and his Ph.D. degree at University of Wuerzburg, Germany, within the PhD program of Elite Network of Bavaria, 2009.
Since 2009, he is a research fellow at Czech Technical University in Prague, where he founded and heads the Multi-robot Systems lab and co-founded Center for Robotics and Autonomous Systems with more than 40 researchers cooperating in robotics.
He was a visiting scholar at University of Illinois at Urbana-Champaign, USA in 2008, and at University of Pennsylvania, USA in 2012, 2014 and 2016, where he worked with Vijay Kumar's group within GRASP lab.
He is an author or co-author of >100 publications in peer-reviewed conferences with multiple best paper awards and more >20 publications in impacted journals, including IJRR, AURO, JFR, ASC, EJC, with >2000 citations indexed by Scholar and H-index 25.
He led a team composed of CTU in Prague, University of Pennsylvania, University of Lincoln that obtained gold medal in 3-challenge, silver medal in 1-challenge and bronze medal in GRAND-challenge of the MBZIRC 2017 competition in Abu Dhabi (http://mrs.felk.cvut.cz/projects/mbzirc, http://mbzirc.com/).
Talk abstract: Deployment of large teams of Micro Aerial Vehicles (MAVs) in real-world (outdoor and indoor) conditions without precise external localisation, such as GNSS and motion capture systems, is a challenging task.
In this talk, I will present the latest results of our endeavor towards fully autonomous compact flocks of MAVs with onboard artificial intelligence.
Stabilization, control, and motion planning techniques for steering swarms of unmanned MAVs will be discussed with the main focus given to biologically inspired techniques that integrate swarming abilities of individual particles with a Model Predictive Control (MPC) methodology respecting the fast dynamics of unmanned quadrotors.
The proposed swarming system relies on a unique sensor of relative localisation of group members using UV-based active markers, which provide a high robustness and reliability in real-world conditions.
Three application scenarios will be discussed and demonstrated in hardware experiments of cooperating MAVs.
1) Compact MAV swarms in high obstacle density areas, such as forest.
2) Indoor documentation of large historical objects (cathedrals) by cooperating MAVs, where one MAV carries a camera and its neighbors carry light sources.
3) Fully autonomous flying robot, Eagle.one, hunting for unauthorized drones that are relatively localised by its onboard sensors.
Yongnan Jia
Lecturer at the School of Automation and Electrical Engineering at the University of Sciences and Technology Beijing, China.
Talk title: Modelling hierarchical flocking
Short bio: Yongnan Jia is currently a lecturer in the School of Automation and Electrical Engineering, University of Sciences and Technology Beijing, Beijing, China. She received her Ph.D. degree in Intelligent Control Laboratory, College of Engineering, Peking University in 2014. She worked as an engineer in the Institute of UAV technology in 2014-2016. She got a post-doctoral position (cooperate with Professor Tamas Vicsek) from Department of Biological Physics, Eotvos University in 2017-2018. Her current research interests include intelligent biomimetic robots, formation control of multi-agent system, cooperation control of manned/unmanned aerial vehicles, and hierarchical flocking.
Talk abstract: We present a general framework for modeling a wide selection of flocking scenarios under free boundary conditions.
Several variants have been considered - including examples for the widely observed behavior of hierarchically interacting units. The models we have simulated correspond to classes of various realistic situations. Our primary goal was to investigate the stability of a flock in the presence of noise. Some of our findings are counter-intuitive in the first approximation, e.g., if the hierarchy is based purely on dominance (an uneven contribution of the neighbors to the decision about the direction of flight of a given individual) the flock is more prone to loose coherence due to perturbations even when a comparison with the standard egalitarian flock is made. Thus, we concentrated on building models based on leader-follower relationships. And, indeed, our findings support the concept that hierarchical organization can be very efficient in important practical cases, especially if the leader-follower interactions (corresponding to an underlying directed network of interactions) have several levels.
Efficiency here is associated with remaining stable (coherent and cohesive) even in cases when collective motion is destroyed by random perturbations.
The framework we present allows the study of several further complex interactions among the members of flocking agents.
Shaojie Shen
Assistant Professor, Dept. of Electronic & Computer Engineering, Hong-Kong University of Science and Technology (HKUST)
Director, HKUST-DJI Joint Innovation Laboratory
Talk title: Building a Complete Aerial Swarm System without External Infrastructure
Short bio:
Shaojie Shen received B.Eng. degree in Electronic Engineering from the Hong Kong University of Science and Technology (HKUST) in 2009.
He received M.S. in Robotics and Ph.D. in Electrical and Systems Engineering in 2011 and 2014, respectively, all from the University of Pennsylvania.
He joined the Department of Electronic and Computer Engineering at the HKUST in September 2014 as an Assistant Professor. He is the founding director of the HKUST-DJI Joint Innovation Laboratory (HDJI Lab).
His research interests are in the areas of robotics and unmanned aerial vehicles, with focus on state estimation, sensor fusion, localization and mapping, and autonomous navigation in complex environments.
He is currently serving as associate editors for T-RO and AURO. His recent work, VINS-Mono, received the Honorable Mention status for the 2018 T-RO Best Paper Award.
He and his research team also won the Best Student Paper Award in IROS 2018, Best Service Robot Paper Finalist in ICRA 2017, Best Paper Finalist in ICRA 2011, and Best Paper Awards in SSRR 2016 and SSRR 2015.
Talk abstract:
Despite recent advances in the autonomy pipeline for aerial robots, the development of aerial robots has reached a stage that the capacity of an individual robot is significantly limited by battery capacities, payload limitations, and sensing constraints. It is therefore desirable to deploy a fleet of aerial robots in real-world applications that requires fast response and coverage, such as surveillance, and search and rescue. In such missions, it is often hard to pre-construct the localization and perception infrastructure for the aerial robot fleet. The problem can be even challenging due to the possibly lack of GNSS reception in complex environments. To this end, we will present our recent results on utilizing multiple sensing modalities to form a decentralized relative localization and navigation framework for aerial swarms. The proposed system is built on top of our robust single robot platform with indoor localization, mapping, and autonomous navigation capabilities.
Each robot is equipped with onboard visual-inertial SLAM with sharable maps, a deep learning-based drone detector, and UWB-based ranging and communication module.
All information is fused in a nonlinear optimization framework to achieve centimeter-level relative localization accuracy in complex indoor environments.
The system features self-configured network topologies, and is robust to temporary loss of a subset of its multiple sensing modalities.
We present real-world experimental results to validate the system performance.
Guido de Croon
Associate professor at the Delft University of Technology
Talk title: Towards swarms of small flying robots in the real world
Short bio: Guido de Croon received his M.Sc. and Ph.D. in the field of Artificial Intelligence (AI) at Maastricht University, the Netherlands.
His research interest lies with computationally efficient algorithms for robot autonomy, with an emphasis on bio-inspired methods for robotic vision and learning.
Since 2008 he has worked on algorithms for achieving autonomous flight with small and light-weight flying robots, such as the DelFly flapping wing MAV. In 2011-2012, he was a research fellow in the Advanced Concepts Team of the European Space Agency, where he studied topics such as optical flow based control algorithms for extraterrestrial landing scenarios.
Currently, he is associate professor at TU Delft and scientific lead of the Micro Air Vehicle lab (MAV-lab) of Delft University of Technology, where he works with his group towards swarms of autonomously flying tiny robots.
Talk abstract:
Swarm robotics holds great potential for real-world applications, promising the swift and robust execution of tasks that would be difficult to achieve by a single robot.
Small flying robots in principle lend themselves well to this concept.
A single, light-weight flying robot is extremely limited in terms of the sensors and processing that can be carried onboard, while successfully flying around requires continuous, quick reactions to the environment.
In this presentation, I will discuss the work I performed with my group on the way to realizing a group of light-weight (< 50g) flying robots that are to explore an unknown environment for exploration missions.
I will delve into the type of artificial intelligence that we give to the robots, and how we achieved to let them sense where neighboring robots are. Sensing other robots in a local neighborhood is a very common and fundamental assumption of much of the theoretical work in the area of swarm algorithms, but is very hard to achieve on small flying robots.
Finally, I will discuss the major remaining challenges towards realizing autonomous swarm exploration.
Wolfgang Hönig
Postdoctoral Researcher at the California Institute of Technology (Caltech).
Talk title: Crazyswarm: A safe and low-cost solution for aerial swarm research
Short bio: Wolfgang Hönig is a postdoctoral scholar at the California Institute of Technology. He holds a Ph.D. in Computer Science from the the University of Southern California (USC), a Diploma in Computer Science from the Technical University Dresden, Germany, and an M.S. in Computer Science (Intelligent Robotics) from USC.
His research focuses on enabling large teams of physical robots to collaboratively solve real-world tasks, combining methods from artificial intelligence and robotics.
Talk abstract: The Crazyswarm is an open-source framework for flying a swarm of quadrotors using the Bitcraze Crazyflie 2.X platform as a control board. The hardware is commercially available and in particular suitable for research purposes in lab settings due to its small size and weight. First, I will discuss the overall system architecture, which enables flying many (40+) quadrotors including state estimation, planning, communication, control, and software tools. Second, I will provide examples on how to use the Crazyswarm for centralized algorithms such as motion planning and decentralized algorithms such as flocking. Finally, I will present an overview of the diverse research projects that have been developed with the Crazyswarm since its first release in 2017.
© 2019 EPFL, all rights reserved